Rachel Porter
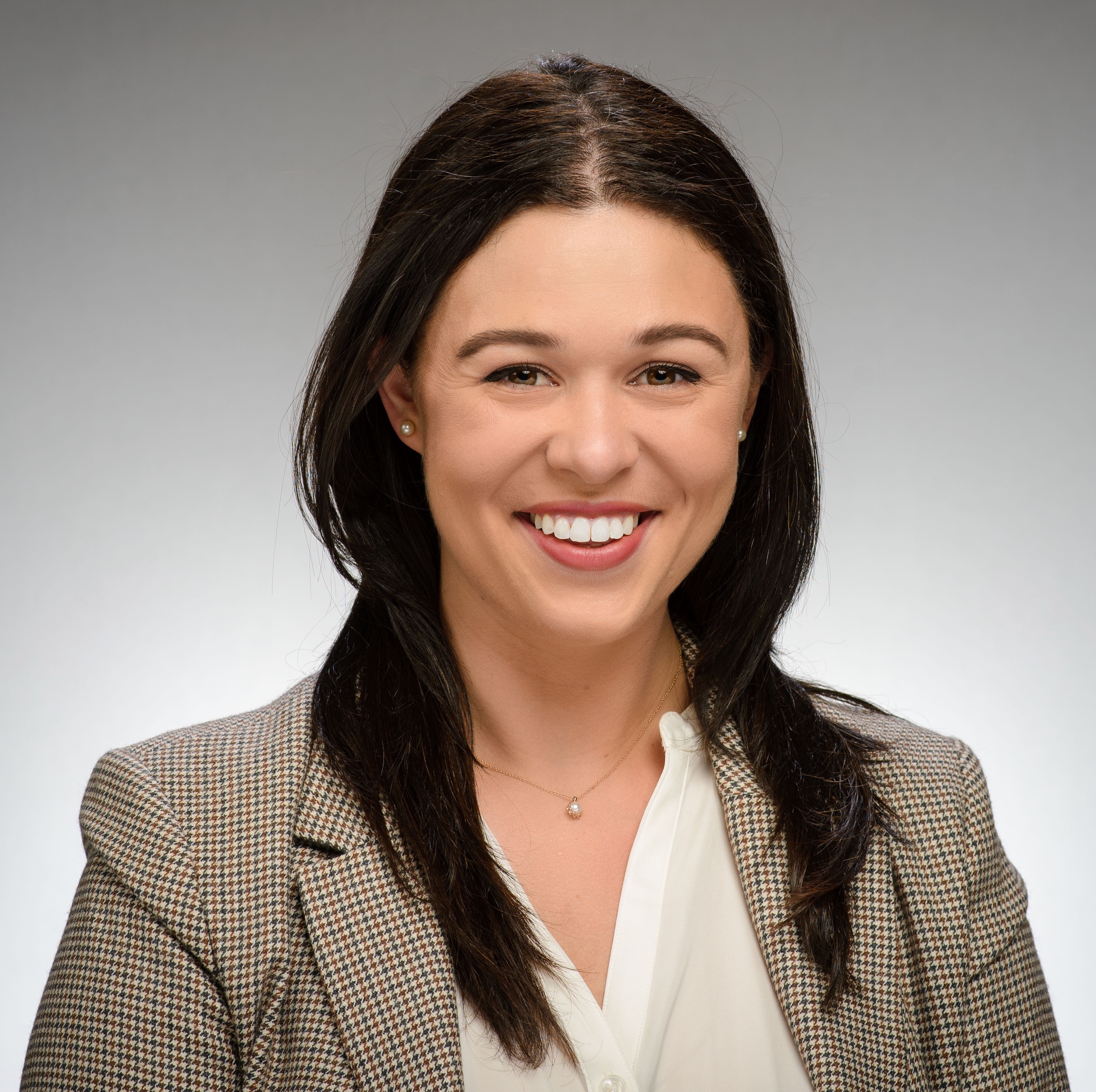
Welcome! I am the Notre Dame du Lac Assistant Professor of Political Science at the University of Notre Dame and a Computational Social Science Faculty Fellow with the Lucy Family Institute for Data and Society. Additionally, I co-direct the Representation and Politics in Legislatures Lab within the Rooney Center for American Democracy. I manage multiple large-scale data collection projects, including CampaignView. If you have any questions about my open data initiatives, please feel free to reach out!
My research centers on American political institutions, with a particular focus on legislative politics, elections, and campaign finance. Broadly, I pair original data collections with tools for natural language processing, machine learning, and causal inference to explore how electoral incentives and institutional design impact political representation.
I received my PhD in 2022 from the University of North Carolina at Chapel Hill. My dissertation, Some Politics Are Still Local: Strategic Position Taking in Congress & Elections, was awarded APSA’s E.E. Schattschneider Award for the best dissertation on American government. My research is published or forthcoming in outlets such as the American Journal of Political Science, The Journal of Politics, and Legislative Studies Quarterly. Additionally, I have a project under contract with Cambridge University Press’s Elements in Quantitative and Computational Methods Series.